Data Analytics for Risk Hedging: A Quantitative Approach for Banking and Financial Institutions to Master Risk Management
The banking and financial sector is a complex and dynamic environment characterized by numerous risks that can severely impact the financial stability and resilience of financial institutions Risk management is therefore a key function within the industry that requires the implementation of effective and efficient risk management strategies.
In our last article of the hashtag#Data_Analytics_for_Risk_Hedging series on The FinData Lake newsletter, we discussed why fraud management is essential for protecting financial institutions from losses, maintaining customer trust, complying with regulations, and managing reputational risk, and explained various machine learning models that might be incorporated within the risk management strategy to mitigate fraud risk and prevent it.
This article, on the other hand, will examine a variety of quantitative methods, scenario analysis, expert judgment, and communication and collaboration techniques that are considered best practices for risk management in banking and the financial sector.
In addition, we will look at the application of these practices through real-life case studies to demonstrate their effectiveness This white paper provides a comprehensive understanding of various risk management strategies and provides financial professionals, business students, and anyone interested in the complexities of the industry with the current financial landscape. It aims to provide insights to effectively manage risk in the current financial landscape.
The following seven points will make you double-think your current risk management strategy, ✒️Take your notes and assess your risks with caution!
1️⃣ 📃Utilizing a combination of quantitative methods for comprehensive risk assessment
Effective risk management is vital in the banking and financial industry. Institutions are constantly exposed to a variety of risks such as credit, market, and operational risks, failing to manage these risks can lead to financial losses, damage to reputation, and even failure of the institution.
Quantitative methods such as statistical modeling, simulation, and machine learning have become increasingly important in managing risk in the banking and financial industry. These methods enable institutions to analyze large and complex data sets, identify patterns and trends, and make informed decisions about risk management.
For instance, statistical models can be utilized to predict risk scenarios and test the resilience of a financial institution’s portfolio, machine learning techniques can be used to identify patterns in data, and Monte Carlo simulations can be used to estimate the potential impact of market events like interest rate changes or credit crises.
In summary, using quantitative methods is crucial in managing risk in the banking and financial industry and ensuring the stability and resilience of financial institutions.
2️⃣ 🔍Incorporating scenario analysis to identify potential risks
Quantitative techniques such as statistical models and simulations are essential for predicting potential risks and assessing the impact of various events on financial institutions’ portfolios. These techniques enable financial institutions to quantify the risks they face and make data-driven risk management decisions.
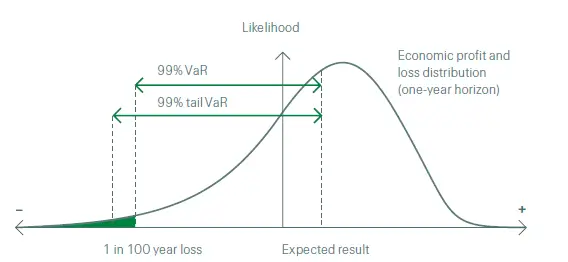
A widely used statistical model in risk management is the Value at Risk (VaR) model. A VaR model calculates the potential loss of a portfolio of financial assets over a specified time frame and confidence level.
For example, a bank can use its VaR model to calculate the potential loss of its portfolio of loans with a 1-day time horizon and a 99% confidence level. You can use it to set risk limits and manage your loan portfolio.
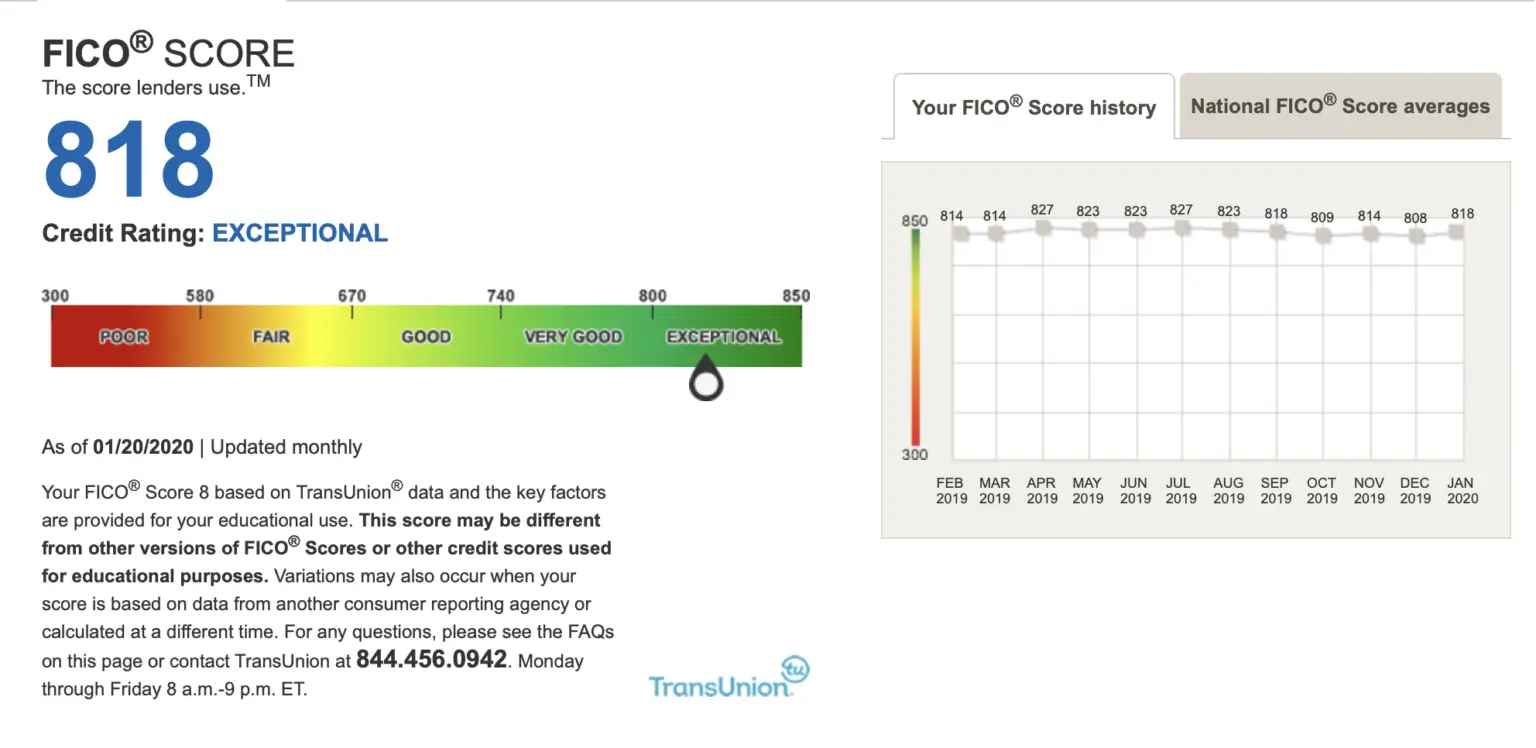
Another common statistical model used in risk management is the Credit Scoring Model. It uses statistical techniques to predict the probability of loan default based on the borrower’s credit history and financial information. Banks and other financial institutions use these models to assess the creditworthiness of potential borrowers and make lending decisions.
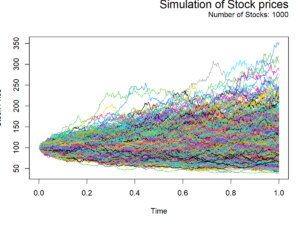
Another example of simulations such as Monte Carlo Simulations can be used to generate multiple random scenarios and estimate the likelihood of different outcomes. Banks and other financial institutions use Monte Carlo simulations to stress test their portfolios to assess the potential impact of market events such as interest rate changes and credit crunch.
The use of quantitative techniques such as statistical models and simulations in risk management enables financial institutions to better understand and quantify risk, leading to more informed risk management decisions.
3️⃣ 🤖Using machine learning techniques to identify patterns and trends in data
Machine learning is a powerful tool for identifying patterns and trends in large amounts of data that can be used as the basis for risk management decisions Machine learning techniques can be applied to a wide variety of data, including financial transaction data, credit bureau data, and market data.
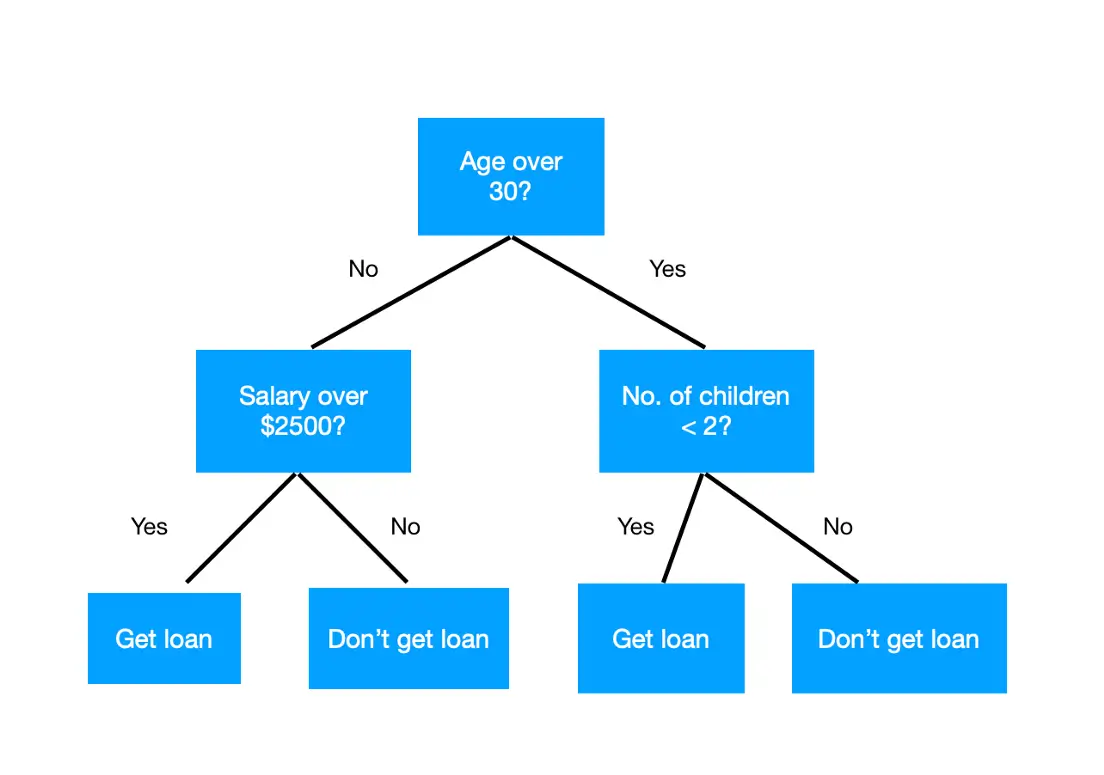
A common machine learning technique used in risk management is the Decision Tree; A decision tree is a type of algorithm that can be used to identify patterns in data and predict future events.
For example, banks can use decision trees to predict the probability of loan default based on a borrower’s credit history and other financial information.
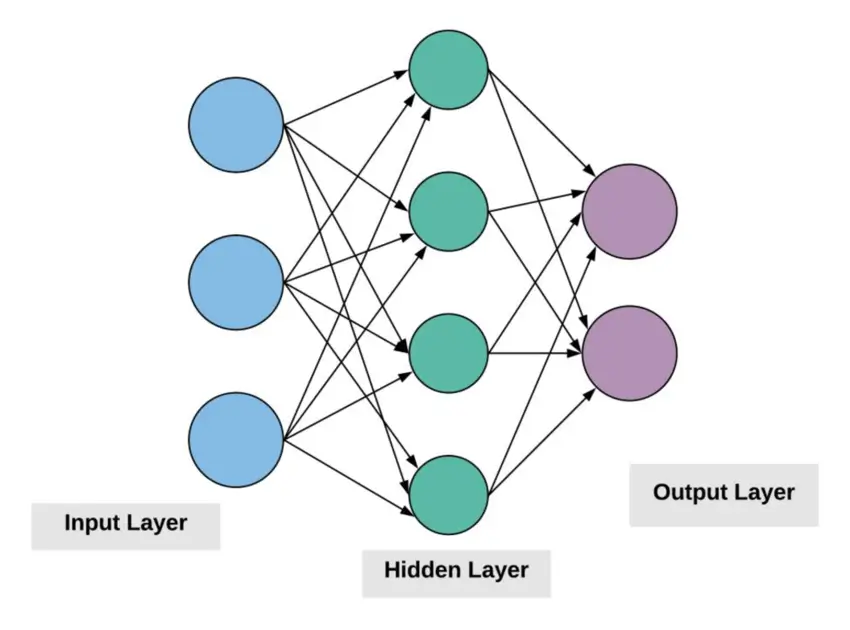
Another machine learning technique used in risk management is Neural Networks; A neural network is a type of algorithm that can be used to identify patterns in data and predict future events They are especially useful when you have a lot of data and the important characteristics are not clear.
Using machine learning techniques for risk management enables financial institutions to analyze large and complex data sets, identify patterns and trends, and make more informed risk management decisions. This will enable more accurate risk assessment and better decision-making, ultimately increasing the resilience of financial institutions.
4️⃣ 📈Emphasizing the need for the right data and infrastructure
Quantitative techniques such as statistical modeling, simulation, and machine learning can be powerful tools for assessing and managing risk in the banking and financial industry, but they require the right data and infrastructure to be effective is required. This means that financial institutions need access to high-quality, relevant data, as well as the resources and expertise to process and analyze that data.
For example, in order to effectively use statistical models that we explained earlier, such as VaR and credit scoring models, financial institutions need accurate and complete data on their asset and liability portfolios. Similarly, the effective use of machine learning techniques by financial institutions requires large and diverse datasets to train the algorithms.
Additionally, financial institutions must have a suitable infrastructure to support the use of quantitative techniques. This includes the hardware, software, and personnel required to process and analyze large amounts of data.
Overall, it is important to ensure that financial institutions have the right data and infrastructure for the effective use of quantitative risk management techniques. Without the right data and infrastructure, the potential benefits of these methods may not be fully realized
5️⃣ 👓Ongoing monitoring and validation through backtesting and stress testing
Quantitative methods can be powerful tools for assessing and managing risk in the banking and financial industry, but it is important to continuously monitor and validate their performance over time This is because financial markets and the risk environment are constantly changing, and what worked well in the past may not be effective in the future.
An important aspect of continuous monitoring and validation is backtesting Backtesting is the process of using historical data to test a quantitative model or strategy. By comparing the model’s predicted results to the actual results, financial institutions can assess the model’s performance and identify weaknesses and areas for improvement.
Another important aspect of continuous monitoring and validation is stress testing Stress testing is the process of simulating extreme market scenarios to assess their potential impact on a financial institution’s portfolio; By simulating different scenarios, financial institutions can identify potential risks and vulnerabilities in their portfolios and take steps to mitigate those risks.
Ongoing monitoring and validation of quantitative risk management techniques are critical to ensuring that these techniques remain effective over time and enable financial institutions to identify and manage risk in changing market conditions.
6️⃣ 🔗Integrating quantitative methods with other risk management practices
Although effective in assessing and managing risk in the banking and financial industry, quantitative techniques such as statistical modeling, simulation, and machine learning should not be the only approach Instead, they should be integrated with other risk management techniques such as scenario analysis and expert judgment.
Scenario analysis is a key risk management strategy that identifies potential risks and assesses their impact on financial institutions This may include identifying market events such as interest rate changes or credit crunch and determining their impact on financial institutions’ portfolios.
Expert opinion, on the other hand, draws on the knowledge and experience of people with specific expertise in risk management. This complements the insights gained from quantitative techniques, providing insight into the likelihood of a particular event or interpreting the results of quantitative models.
By integrating quantitative techniques with other risk management techniques, financial institutions can gain a more comprehensive understanding of risk and make more informed risk management decisions. Moreover, combining the strengths of different methodologies and perspectives can help financial institutions create a more robust and resilient risk management framework.
7️⃣ 🧑🤝🧑Importance of ongoing communication and collaboration among departments
Effectively implement and use quantitative techniques in risk management, emphasizing the importance of continuous communication and collaboration between various departments within a financial institution.
The implementation and application of quantitative methods in risk management require close cooperation and communication between various departments within financial institutions. This includes collaboration between risk management, finance, and technology departments, and communication between front and back office staff.
For example, the risk management department is responsible for identifying and evaluating potential risks, while the finance department is responsible for implementing and overseeing risk management strategies Technical departments, on the other hand, are responsible for providing the necessary infrastructure and support for the use of quantitative methods In addition, front office staff such as traders and portfolio managers should be aware of the risks associated with their activities and how to manage them.
Ongoing communication and collaboration between various departments within a financial institution are essential to the effective implementation and use of quantitative techniques in risk management. By working together and sharing information, different departments can more fully understand the risks of a financial institution and make more informed risk management decisions.
🛫 The Takeaway
In summary, effective use of quantitative techniques for risk management in banks and financial institutions is key to:
- Use a combination of various quantitative techniques such as statistical modeling, simulation, and optimization to gain a more comprehensive understanding of risk.
- Use scenario analysis to identify potential risks and assess their potential impact on financial institutions.
- Use machine learning techniques such as decision trees, neural networks, and natural language processing to identify patterns and trends in large data sets that can be used as the basis for risk management decisions.
- Ensure that the right data and infrastructure are in place to effectively use quantitative techniques in risk management.
- Continuously monitor and validate the performance of quantitative methods over time through backtesting and stress testing.
- Integrate quantitative techniques with other risk management techniques, such as scenario analysis and expert judgment.
- Facilitate ongoing communication and collaboration between various departments within a financial institution and effectively implements and use quantitative techniques in risk management.