Natural Language Processing (NLP) and Generative Applications for Risk Management in Banking and Financial Institutions
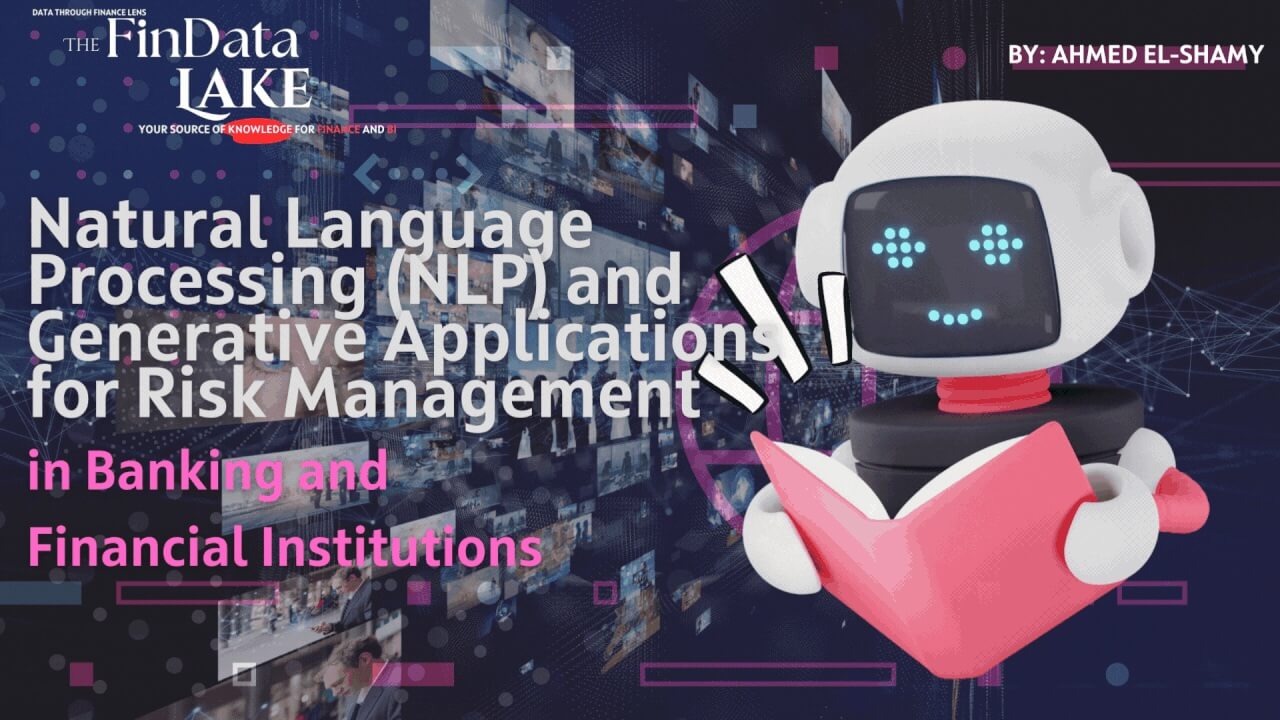
Introduction
Managing risks effectively is crucial for ensuring stability and success. In order to achieve such milestone, multiple factors must be considered. One of the most transformative technologies driving risk management forward is Natural Language Processing (NLP) and Generative Applications. This article explores the significant impact of NLP and generative models in risk management processes, from sentiment analysis to fraud detection, automated report generation, and more.

2.1 👀 What is NLP?
NLP is a branch of artificial intelligence (AI) that focuses on the interaction between computers and human language. It enables machines to understand, interpret, and generate human language, making it a powerful tool for processing vast amounts of textual data.
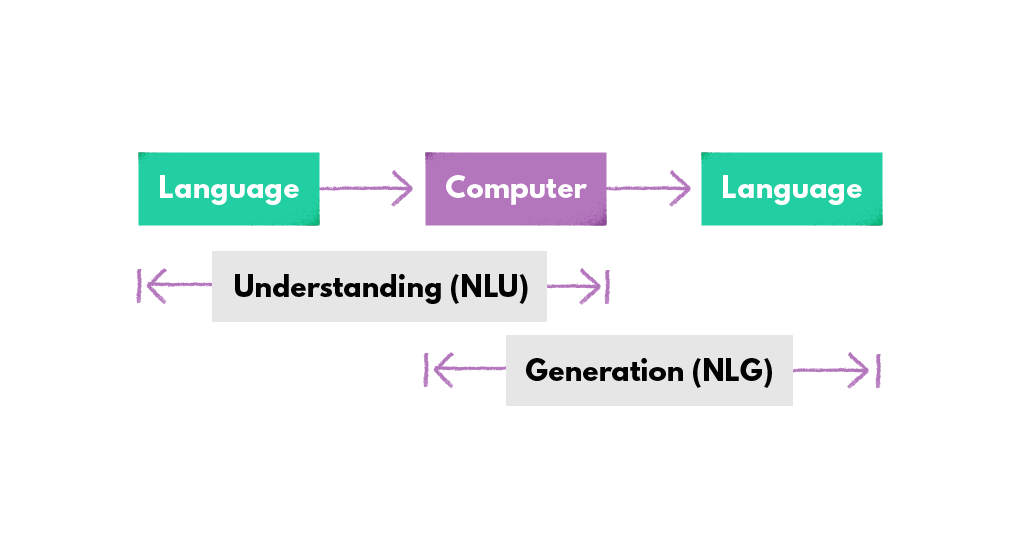
2.2 💡 Importance of NLP in Risk Management
In risk management, access to relevant information is vital. NLP allows financial institutions to analyze unstructured data, such as news articles, social media, and customer feedback, to extract valuable insights. These insights contribute to making informed decisions and mitigating potential risks effectively.
By 2025, almost 30% of the applications of Natural Language Processing will be carried out inside Banking, Financial Services, and Insurance. Banking has historically been the natural promoter of the application of AI, more specifically, NLP for finance, in data automation.
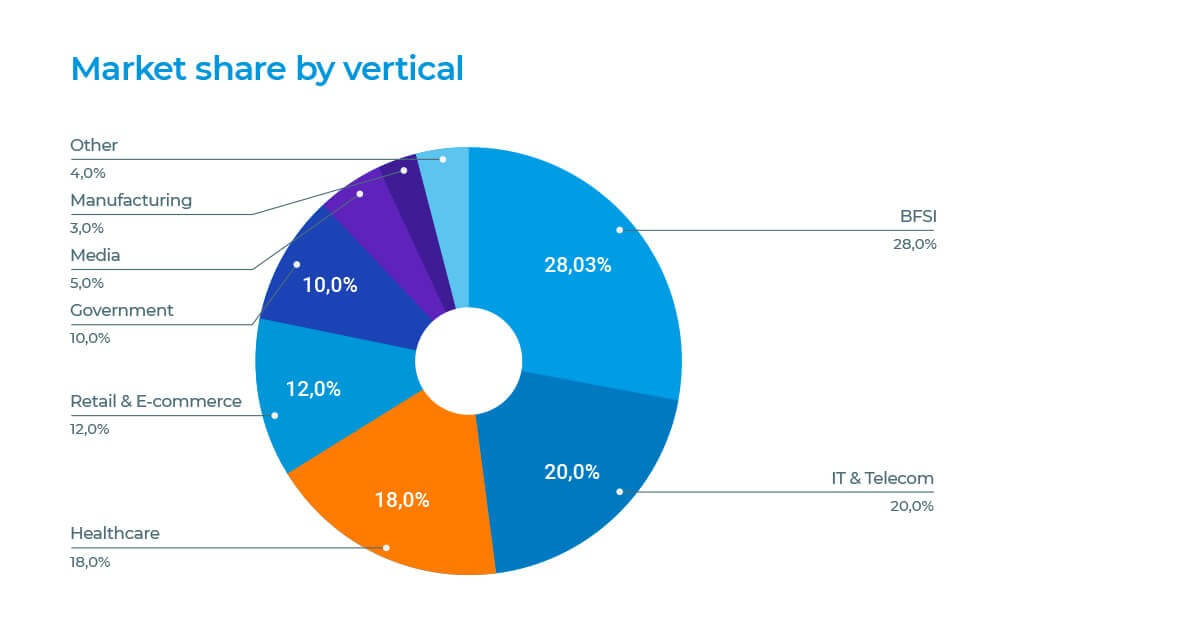
NLP in Finance (BFSI sector) is already used for:
- Automatic loan, credit applications
- Automatic calculations of fees
- Customer onboarding
- Risk Management
- Asset Management, ESG
- Compliance
One may think that NLP will be limited to certain usages. This is completely wrong! NLP usage will revolutionize all banking/non-banking risk sectors; it will allow non-technical users to dive deep into a higher level of analysis/analytics across multiple risk dimensions.
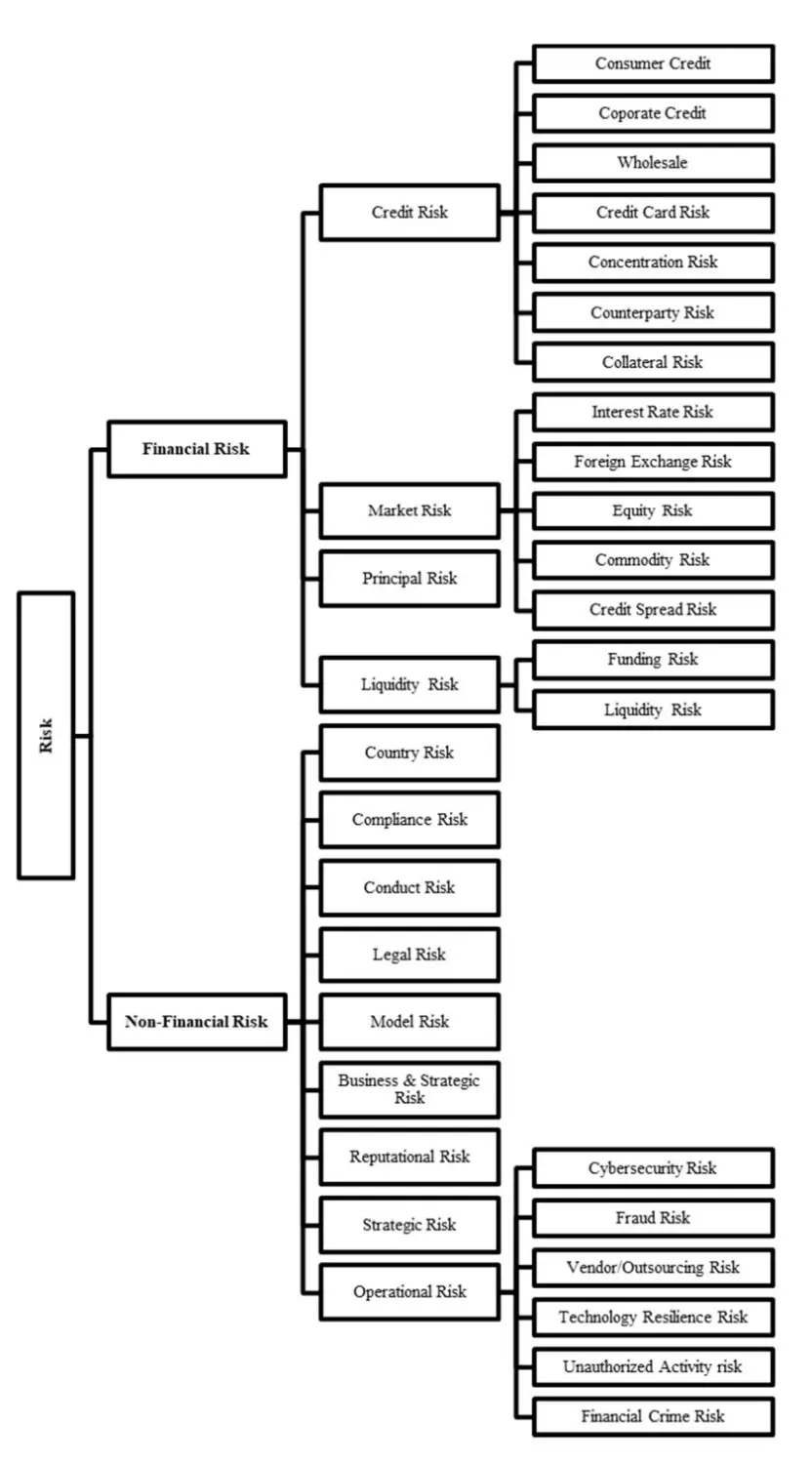

It is safe to say that the usage of NLP & AI into Risk Applications in Banking and Financial Institutions will be implemented into every single section of Risk. The chief risk officer has access to risk insight and intelligence that was more retrospective in nature, such as incident analyses focusing on understanding what happened and why.
Now, increasingly, they are gearing up with tools that allow for a look ahead that facilitates the predicting of potential risk incidents. Data mining, scenario modelling and forecasting are built-in features of most risk management solutions.
Cognitive (pattern recognition by visualising and identifying apparent and later trends in historical data) and algorithmic (establishing causal relationships between diverse events and data sets) intelligence is making way for augmented (natural language processing and machine learning) and assistive (contextual virtual intelligent assistance) intelligence that augments and accelerates decision making.
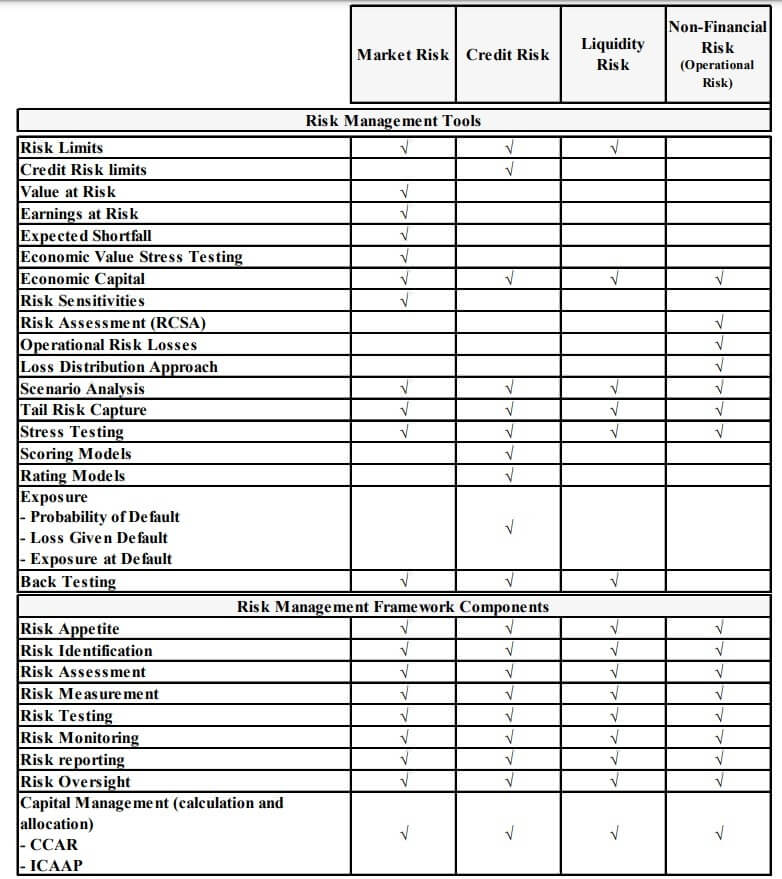
Below, are some of the currently anticipated NLP Application Delivery for Banking and Financial Institutions:
3.1 😁 Sentiment Analysis for Customer Feedback

Customer feedback plays a crucial role in shaping financial products and services. NLP-powered sentiment analysis helps institutions understand customer perceptions and satisfaction levels. Positive sentiments can highlight successful ventures, while negative sentiments can indicate potential issues that require immediate attention.
3.2 🦹🏻♂️ Fraud Detection and Prevention
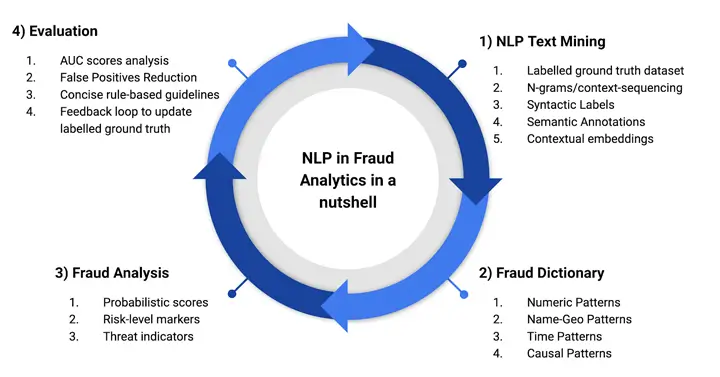
Fraud is a constant concern in the financial industry. NLP algorithms can scrutinize vast amounts of transactional data and identify suspicious patterns or anomalies that indicate fraudulent activities. Utilizing NLP to analyze classified/non-classified big data for Fraud Detection is already here, and many companies are offering such products to be used to combat fraud risks. This capability enables banks to respond promptly and prevent financial losses.
Such capabilities revolve around:
a. NLP Text Mining
The usage of NLP in Fraud Analytics for Text Mining is undervalued. NLP can label ground truth dataset, label various text datasets, and use contextual embeddings.
b. Fraud Dictionary
Detecting patterns is not an easy game for any analysts. but by the usage of NLP in Fraud Analytics for Fraud Dictionary, it’ll help all fraud analysts to derive data-driven decisions based on the current data that they have. It’ll also help them in detecting numeric, name-geo, time and casual patterns.
c. Fraud Analysis
Building KRIs for Fraud Analysis is done on best-efforts-basis. Such thing varies from an institution to another, and has no definitive book to achieve. Using NLP in Fraud Analytics for Fraud Analysis will help directors and managers to establish probabilistic scores, risk-level metrics, and threat (KRI) indicators.
d. Evaluation
At last but not least, NLP in Fraud Analytics will definitely help users to build AUC score analysis, and reduce false positive ratios as well as building rule-based grounds.
3.3 📜 Automated Report Generation
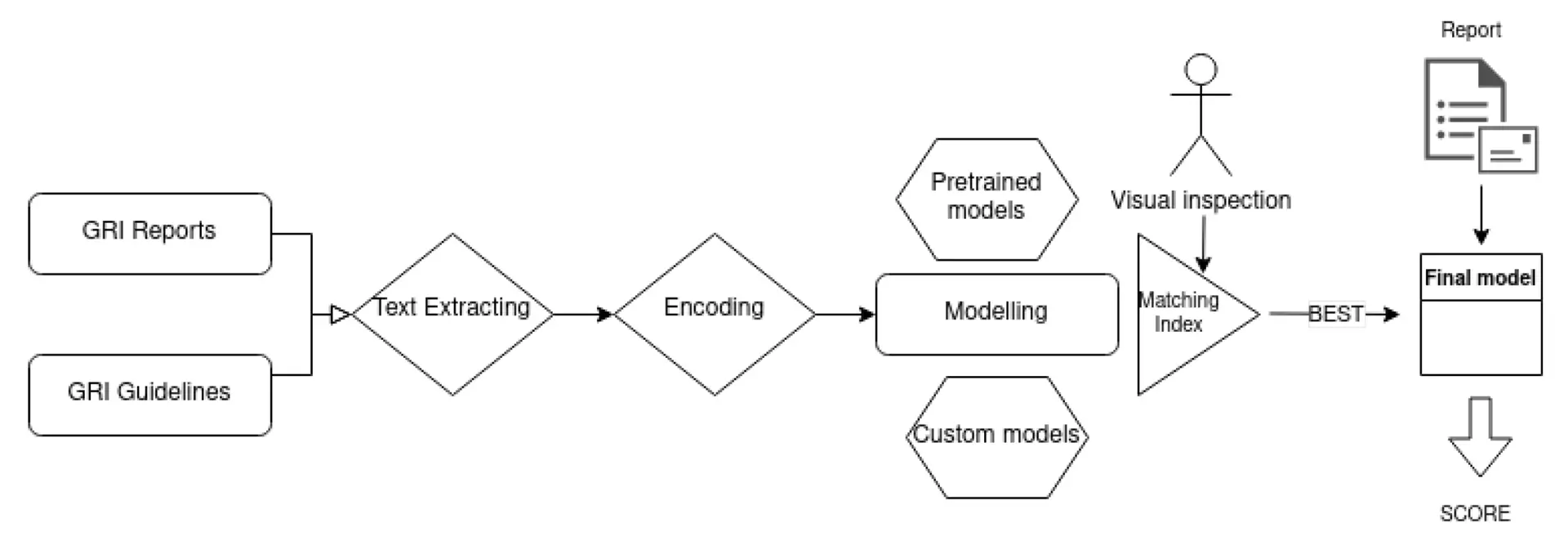
Traditional risk reports can be time-consuming and labor-intensive to create. NLP automates the report generation process, extracting relevant information from various sources and summarizing it into concise reports. This automation saves time, reduces errors, and facilitates better decision-making.
The most famous tool that is used for such task is ChatGPT. You can give it the context of the report, and it’ll generated a detailed report for you within seconds. Saving hours of work and effort to build it.
3.4 🈸 Data Classification using Transformative Applications
Having multiple sources of unclassified data in form of text, image, and video is not a satisfying information to know. Especially when your institution relies on it, heavily! But such thing will be a thing of the past. Using Machine Learning for Transformative Binary Applications such as Financial Image Transformers (ViT) in Spark NLP to detect receipts in both scanned and mobile images will solve it for good. Take a look at the following example:


4.1 💻 Generative Adversarial Networks (GANs)
Generative Adversarial Networks (GANs) are a class of generative models used to create synthetic data. In finance, GANs can generate realistic financial data, such as stock market trends, which can aid risk analysts in stress-testing and scenario planning.
4.2 🚫 Use Cases in Risk Management
GANs can simulate potential market outcomes and assess the impact of various risk factors. Financial institutions can use this capability to devise robust risk management strategies and evaluate their effectiveness in simulated scenarios.

5.1 👨🏻⚖️ Enhanced Decision-Making
The insights derived from NLP analysis and generative models empower risk managers to make data-driven decisions. This leads to more accurate risk assessments and proactive risk management practices.
5.2 ☺️ Improved Customer Experience
By understanding customer sentiments through NLP, financial institutions can tailor their products and services to meet customer expectations, resulting in an enhanced overall customer experience.
5.3 🔍 Real-time Risk Assessment
NLP enables real-time and near-real-time analysis of market trends and emerging risks. Paired with generative applications, institutions can respond promptly to dynamic market conditions and potential threats.

6.1 🔐 Data Privacy and Security Concerns
Using NLP to analyze unstructured data raises data privacy and security concerns. Institutions must implement robust data protection measures to safeguard sensitive information.
6.2 🎯 Ensuring Model Robustness and Accuracy
NLP and generative models heavily rely on the quality and quantity of data. Ensuring the accuracy and robustness of these models is essential to avoid making critical decisions based on flawed information.

7.1 📃 Fairness and Bias in Algorithms
NLP algorithms can inadvertently perpetuate biases present in training data. Institutions must actively address and rectify biases to ensure fair treatment of all customers and stakeholders.
7.2 🧑🏻🏫 Transparency and Explainability
As AI-driven technologies become more sophisticated, explaining the rationale behind their decisions becomes crucial. Institutions must prioritize transparency and provide clear explanations for the outcomes generated by NLP and generative models.

As technology continues to advance, the use of Natural Language Processing (NLP) and Generative Applications in risk management for banking and financial institutions becomes increasingly indispensable. The ability to extract valuable insights from vast amounts of textual data and generate contextually relevant information empowers risk managers to make informed and proactive decisions. Embracing these transformative technologies not only enhances risk assessment processes but also drives the overall efficiency and resilience of the financial sector. As the industry continues to evolve, the adoption of NLP and Generative Applications will undoubtedly be a key differentiator for successful risk management in the future.
🤔 FAQs ⁉️
1. How does NLP help banks in risk management?
NLP enables banks to analyze vast amounts of textual data, extract valuable insights, and make data-driven decisions to manage risks effectively.
2. Can NLP prevent financial fraud?
Yes, NLP can aid in fraud detection by analyzing transactional data and identifying suspicious patterns indicative of fraudulent activities.
3. What are Generative Adversarial Networks (GANs) in finance?
GANs are generative models that can create synthetic financial data, helping in stress-testing and scenario planning for risk management.
4. Are NLP algorithms biased?
NLP algorithms can be biased if trained on biased data. Institutions must actively address and rectify biases to ensure fairness.
5. What does the future hold for NLP and generative applications?
The future may bring more advanced sentiment analysis, improved fraud detection models, and expanded use of GANs in risk management.
LSI Keywords
- Risk management in banking
- Financial risk assessment
- NLP applications in finance
- Generative models in banking
- Artificial intelligence for risk management
- Sentiment analysis in finance
- Fraud detection using NLP
- Credit risk evaluation
- Automated financial reports
- Scenario modeling in banking
- Stress testing for financial institutions
- Compliance using Generative Applications